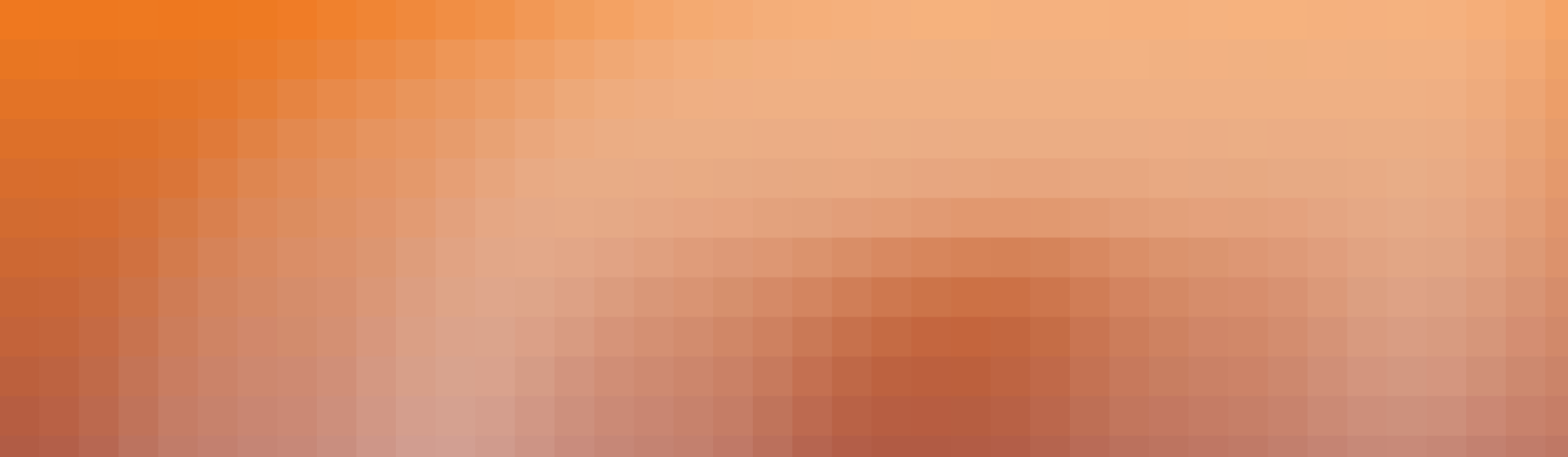
Blog
Discover SqueezeBits team blog
-
OwLite: No More Compromising on AI Performance After Quantization
Discover how OwLite simplifies AI model optimization with seamless integration and secure architecture.
-
[Intel Gaudi] #5. FLUX.1 on Gaudi-2
This article discusses inference efficiency when running the FLUX.1 models on Intel Gaudi-2 hardware.
-
TensorRT-LLM Goes Open Source!
A couple of weeks ago, TensorRT-LLM finally open-sourced most of its code. The released code includes the following.
-
When Should I Use Fits on Chips?
In this article, we explore user cases demonstrating how Fits on Chips enables efficient LLM inference, from tuning models in constrained environments to selecting the best GPU for different workloads and benchmarking quantized models to assess the trade-offs between compression and output quality.
-
Fits on Chips: Saving LLM Costs Became Easier Than Ever
In the following sections, we’ll briefly explore the key factors that affect LLM serving. We’ll also examine how Fits on Chips centralizes and automates parameter exploration, ultimately lowering the barriers to running high-quality LLMs in an organization’s own environment.
-
SLEB: Streamlining LLMs through Redundancy Verification and Elimination of Transformer Blocks
SLEB is a newly introduced efficient pruning method with the goal of optimizing LLMs without compromising linguistic prowess.
-
The Missing Piece of TensorRT-LLM
In this post, we share our approach — Direct Torch to TensorRT-LLM Optimizer (Ditto)— to streamlining the TensorRT-LLM's model conversion by leveraging PyTorch's modern compilation stack, specifically via torch.export and Torch-TensorRT.
-
The Rise and Fall of ONNX (feat. PyTorch 2.0)
In this post, we will journey through ONNX’s lifecycle: from its revolutionary inception during the computer vision boom to its gradual fade as more integrated solutions emerged, and finally, to its current status as a specialized yet indispensable tool in certain scenarios.
-
[vLLM vs TensorRT-LLM] #13. Vision-Language Models
In this blog post, we compare the serving performance of two leading frameworks—vLLM and TensorRT-LLM—using LLaVA-1.5-7B-HF, one of the most popular model with vision and language capabilities.
-
[Intel Gaudi] #4. FP8 Quantization
In this post, we will focus on one of Gaudi-2's key advantages over the A100: support for the FP8 format.
-
[Intel Gaudi] #3. Performance Evaluation with SynapseAI v1.19
In this post, we will present the updated performance metrics for Gaudi-2 in large language model (LLM) inference and discuss the enhancements that contributed to this significant improvement.
-
[vLLM vs TensorRT-LLM] #12. Automatic Prefix Caching
This article provides a comparative analysis of automatic prefix caching.
-
[vLLM vs TensorRT-LLM] #11. Speculative Decoding
This article provides a comparative analysis of speculative decoding.
-
[vLLM vs TensorRT-LLM] #10 Serving Multiple LoRAs at Once
This article provides a comparative analysis of multi-LoRA serving capabilities of vLLM and TensorRT-LLM frameworks.
-
[Intel Gaudi] #2. Graph Compiler and Overall Performance Evaluation
In this post, we will explore the intricacies of the Graph Compiler and its impact on LLM serving frameworks.
-
[vLLM vs TensorRT-LLM] #9. Parallelism Strategies
This article provides a comparative analysis of different parallelism strategies on vLLM and TensorRT-LLM frameworks.
-
[Intel Gaudi] #1. Introduction
In this blog series, we thoroughly evaluate Intel's AI accelerator, the Gaudi series, focusing on its performance, features, and usability.
-
[vLLM vs TensorRT-LLM] #8. KV Cache Quantization
This article provides a comparative analysis of the effects of KV cache quantization on vLLM and TensorRT-LLM frameworks.
-
[vLLM vs TensorRT-LLM] #7. Weight-Activation Quantization
This article provides a comparative analysis of the effects of weight-activation quantization on vLLM and TensorRT-LLM frameworks.
-
[vLLM vs TensorRT-LLM] #6. Weight-Only Quantization
This article provides a comparative analysis of the effects of weight-only quantization on vLLM and TensorRT-LLM frameworks.
-
[vLLM vs TensorRT-LLM] #5 Dynamic Sequence Lengths
This article provides a comparative analysis of vLLM and TensorRT-LLM frameworks, focusing on performance with fixed and dynamic datasets.
-
[vLLM vs TensorRT-LLM] #4 Which Scheduler Wins?
The era of Transformers and Large Language Models (LLMs) is flourishing.
In this post, we will explore how these schedulers function and how they affect performance and resource utilization. -
[vLLM vs TensorRT-LLM] #3 Understanding Sampling Methods and Their Performance Impact
In this article, we will start by exploring key sampling techniques: Top-K, Top-P, and repetition penalty. Then, we will assess the performance overhead of these techniques under different configurations on both the TensorRT-LLM and vLLM frameworks.
-
[vLLM vs TensorRT-LLM] #2. Towards Optimal Batching for LLM Serving
In this article of our series, we explore deeper by tuning key parameters such as maximum batch size and maximum number of tokens. We will adjust these parameters step by step to investigate how they impact the performance of each framework.
-
[vLLM vs TensorRT-LLM] #1. An Overall Evaluation
This article provides a comparative analysis of vLLM and TensorRT-LLM frameworks for serving LLMs, evaluating their performance based on key metrics like throughput, TTFT, and TPOT to offer insights for practitioners in optimizing LLM deployment strategies.
-
OwLite로 YOLOv5 모델 경량화하기 3 - QAT, Dynamic batch size 엔진 생성
QAT(Quantization Aware Training)는 양자화를 고려하여 학습하는 방법으로, PTQ(Post-Training Quantization) 적용 시 발생할 수 있는 정확도 손실을 다시 복구하기 위해 사용됩니다. 이를 통해 단순히 PTQ를 적용할 때보다 더 높은 정확도를 유지하면서도 모델을 경량화 할 수 있는 장점이 있습니다. 그래서 이번 편에서는 PTQ 후 추가로 QAT를 적용하여 mAP를 더욱 향상시키는 방법을 살펴보려고 합니다.
-
OwLite로 YOLOv5 모델 경량화하기 2 - Experiment 생성, Quantization option 적용하기
스퀴즈비츠에서 출시한 AI 경량화 툴킷인 OwLite는 AI 모델을 간편하게 경량화할 수 있도록 도와드립니다. 1편에 이어 OwLite를 활용하여 YOLOv5 모델을 경량화하는 과정을 소개할 예정인데요, 이번 글에서는 등록한 Baseline 모델에 다양한 경량화 옵션을 적용하고 가장 빠른 모델을 선택하는 과정을 보여드리겠습니다.
-
OwLite로 YOLOv5 모델 경량화하기 1 - OwLite 환경 세팅, Baseline 등록
이번 글에서는 가상의 고객이 OwLite로 AI 모델을 경량화하는 과정을 통해 OwLite를 실제 모델에 적용하는 방법과 이를 통해 얻을 수 있는 성과를 보여드리고자 합니다. OwLite를 실제 작업에 적용하면서 마주할 수 있는 다양한 상황들을 함께 살펴보시죠!
“Edge device에서 Object Detection AI를 사용해야 하는데, YoloV5 모델의 inference에 걸리는 시간이 너무 길어 실시간 서비스가 어렵습니다.”
-
스퀴즈비츠 브랜딩 가이드 구축기
최근 스퀴즈비츠 홈페이지가 리뉴얼된 것을 눈치채셨나요? 스퀴즈비츠의 상징이었던 주황색에 더해, 보라색이 추가된 그래픽으로 홈페이지가 한층 더 다채로워졌습니다. 오늘은 스퀴즈비츠 홈페이지를 리뉴얼하는 과정 속 브랜딩 가이드를 구축하게 된 이유와 그 절차에 대해 이야기해 보고자 합니다.
리뉴얼을 고민하면서 ‘우리를 어떻게 잘 설명할 수 있을까?’와 ‘우리는 누구이며, 어디로 나아가고 있는가?’라는 질문에 직면하게 되었고, 이 과정에서 좋은 웹사이트를 만들기 위해서는 브랜딩이 우선되어야 한다는 결론에 도달했습니다.
-
[EN] FP8 Quantization with OwLite
Over the past few years, AI has made tremendous progress, and applications based on large language models (LLMs), such as ChatGPT, have already helped us in various areas of our lives. This progress can be attributed to the development of deep learning algorithms, the exponential increase in data, and groundbreaking computing power advances. In this context, new data representation formats have emerged, enabling efficient data processing and model deployment.
-
[KR] OwLite와 함께하는 FP8 Quantization
지난 몇 년간 AI 분야는 엄청난 발전을 이루었고, ChatGPT와 같은 대규모 언어 모델(LLM) 기반의 어플리케이션은 이미 다양한 영역에서 우리 삶 속에 스며들어 큰 도움을 주고 있습니다. 딥러닝 알고리즘의 발전, 폭발적으로 증가하는 데이터, 그리고 컴퓨팅 파워의 혁신적인 발전이 이러한 성과를 이끌었다고 볼 수 있습니다. 이러한 배경 속에서 효율적인 데이터 처리와 모델 배포를 가능하게 하는 새로운 데이터 표현 방식들이 등장하고 있습니다. 그중에서도 FP8 (Floating-point 8-bit) format은 AI 하드웨어 아키텍처에서 주목할 만한 혁신 중 하나로 떠오르고 있습니다.
-
취업 준비 필수 가이드: 채용 행사 활용하기
일자리박람회, 채용상담회, 채용설명회… 취업이나 이직을 준비하시는 분들이라면 한 번 쯤은 관심을 가져보시거나 참가해 보셨을 채용 관련 행사들입니다.
저도 첫 취업을 준비하던 시절엔 이런저런 행사들을 많이 검색해 보고 찾아다니기도 했습니다. 동시에 어떤 마음가짐과 질문 리스트를 가지고 참가해야 할지 걱정이 많이 되기도 했어요. 오늘은 스퀴즈비츠가 최근 참가했던 채용 행사들을 간략하게 소개해 보며, 채용 상담에 참여하는 분들께서 어떤 질문을 가져가시면 좋을지도 함께 풀어보겠습니다.
-
How much can we save through compression?
Cost reduction in model serving is a major concern for companies that deliver AI-based products and services. The hefty price of AI deployment has made it challenging for companies to budget on inference-related expenses. Most of these expenses arise from heavily using clusters of GPUs. Squeezebits offers cost-effective, practical solutions based on model compression that lift those stressful burdens.
-
현장에서 체험해보는 AI 경량화, IT 전시회 참여기
요즘 각종 전시회들이 참 다양하게 많이 열리고 있습니다. 코로나 팬데믹으로 몇 년간 오프라인 행사에 다들 갈증을 느껴서인지, 코로나 이전보다 행사 건수와 참관객이 더 증가했다는 통계도 있습니다. 나의 관심 분야와 유관한 여러 업체, 상품, 컨텐츠 등을 한 곳에서 둘러볼 수 있다는 점이 전시회의 가장 큰 장점이겠죠. 많은 정보를 한자리에서 수집할 수 있을 뿐만 아니라 직접 눈으로 보고 체험해 볼 수 있기 때문에 많은 분들의 관심이 쏠리는 듯합니다.
-
‘Breaking Down’ Tokenizers in LLMs
Using tokenizers, or tokenization, is the first and fundamental step in the NLP pipeline. It is the process of translating natural language(text input) to an appropriate format(numbers) so that a machine learning model can understand it. Tokenizers break down the text into smaller pieces such as words or characters. These smaller pieces are called ‘tokens.’
-
SLEB: Streamlining LLMs through Redundancy Verification and Elimination of Transformer Blocks
SLEB is a newly introduced efficient pruning method with the goal of optimizing LLMs without compromising linguistic prowess. The SLEB method is distinct from previous methods as it proposes to have the transformer block as the fundamental unit of pruning.
-
Accuracy Degradation in AI Compression: Myth or Truth?
The benefits of neural network compression have been made clear(in our previous posts). As advocates of compression technology, we commend using compression methodology to enhance deep learning models. Yet, many skeptics still hesitate to utilize compression.
-
AI 경량화를 툴킷 하나로, OwLite -2/2
OwLite는 AI 모델을 쉽게 경량화하면서도 강력한 성능을 유지할 수 있는 툴킷입니다.
새롭게 생겨나는 경량화 수요에 대응하기 위해 OwLite는 이전에는 없던 많은 기술적, 기획적 고민을 거쳐 탄생했습니다. 오늘은 OwLite의 출시를 맞아 저희가 걸어온 과정을 소개해 보겠습니다. OwLite가 탄생한 과정과 고민을 알고 나면 OwLite 가 어떤 기능을 가지고 있는지, 여러분들에게 어떤 도움을 드릴 수 있는지 명확해질 것이라고 생각합니다.
-
Are you getting everything out of your GPUs?
At the 2024 GTC event, Nvidia CEO Jensen Huang got on the stage to deliver his keynote speech, in which he divulged the newest GPU generation, the ‘Blackwell.’ In his speech, he claimed that within the last 8 years leading up to Blackwell, AI computation enhancements of 1000 times were achieved thanks to the advancements of the GPU chip.
-
4 Types of AI Compression Methods You Should Know
Our last post discussed the advantages and indispensability of compressing AI models. In this writing, we further explore the idea of AI compression, exploring 4 types of existing compression methodology with the objective of creating succinct and efficient neural networks.
-
Things to check if your business utilizes AI
As the AI revolution quickly reshapes our business landscape, an increasing number of organizations are implementing a state of the art AI model. Even non-tech companies in domains such as healthcare, security and marketing are integrating AI into their business architecture to enhance decision making and further expand business opportunities.
-
AI 경량화를 툴킷 하나로, OwLite -1/2
세상을 뒤덮는 AI 관련 소식을 보면 AI가 본격적으로 우리의 생활에 가까워지고 있다는 실감이 납니다.
다양한 서비스가 AI를 활용해 새로운 사용자 가치를 제공하고자 노력하고 있습니다. AI 역시 IT 서비스를 이루는 다른 구성 요소처럼 비용이나 보안 등 여러 제약에서 자유로울 수 없는데요, 많은 컴퓨팅 리소스를 필요로 하는 AI의 특성상 이러한 문제에 더 민감하다고 할 수 있습니다.
-
AI 경량화를 경험하면서 군복무를 할 수 있다고?
2024년 첫 포스팅은 올해 1월 1일 자로 업데이트된 따끈따끈한 뉴스로 시작해 보려 합니다. 바로 스퀴즈비츠가 병역지정업체에 선정되었다는 소식입니다! [AI 경량화]라는 분야가 높은 전문성과 관심도를 요하는 만큼, 우수한 인재분들을 메리트 있게 모시기 위해 스퀴즈비츠는 재작년부터 발 빠르게 병역지정업체 신청을 준비했는데요. 법인 설립 만 2년도 되지 않은 빠른 시기에 전문연구요원과 산업기능요원 두 부문 모두 선정되어 더더욱 자랑스럽고 신나는 결과랍니다!
-
[복지 제도 소개] 이 회사, 다니고 싶다
이번 포스팅에서는 스퀴즈비츠의 복리후생 제도를 소개 해 드리려 합니다. 여러분께서는 ‘복지 제도가 훌륭한 회사’라면 무엇이 떠오르시나요? 가장 먼저 아낌 없이 지갑을 여는(!) 빵빵한 지원이, 혹은 일하기 편하게 구축된 근무 환경 등이 있겠죠! 스퀴즈비츠 역시 팀원들이 자부심을 느낄 수 있는 제도와 문화를 만들기 위해 참 고심했는데요, 지금부터 스퀴즈비츠 팀원이 누릴 수 있는 모든 것들을 A to Z로 소개해 드리겠습니다!
-
우리의 상반기는 어땠나요
스퀴즈비츠 타운홀 미팅은 팀원들이 ‘우리의 현 위치와 다음 스텝, 그리고 중장기적 비전을 인지하고 내재화한다’는 의미에서 대표님이 직접 진행하신답니다. 이번 타운홀 미팅은 작년 연말 미팅에서 공유되었던 올해의 목표는 얼마나 달성되었는지, 각종 프로젝트는 어떻게 진행되고 있는지, 그뿐만 아니라 투자 실적과 재무 현황까지도 투명하게 공개하는 자리였습니다.
-
Get Study with Me
요즘 인공지능 분야에서는 하루가 다르게 새로운 개념과 기술이 쏟아지고 있습니다. 스퀴즈비츠는 이런 경량화 및 가속화 기술의 최전선에 서기 위해 많은 노력을 기울이고 있습니다.
스퀴즈비츠는 대학원에서 부터 경량화 기법을 연구해오시던 분, 경량화된 AI를 실제 어플리케이션에 배포하시는 분, NPU 회사에서 하드웨어 가속을 하시던 분 등 여러 배경지식을 가진 분들과 함께하고 있습니다.
-
핵심가치, 우리가 추구하는 문화는
조직을 승리로 이끄는 힘의 25%는 실력이고, 나머지 75%는 팀워크라는 말이 있습니다.
스퀴즈비츠라는 팀이 만들어지고 제일 먼저 미션과 핵심가치를 고민했던 이유입니다. 아무리 훌륭한 인재들이 모여있다 해도 우리만의 문화가 세워지지 않으면 충분한 시너지를 낼 수 없습니다. 같은 목표를 바라보고, 같은 가치관을 공유할 때 비로소 최대의 공명이 완성되는 것입니다.
-
스퀴즈비츠 팀 블로그를 소개합니다
팀 블로그는 그 어떤 매체보다도 팀의 모습을 생생하게 드러낼 수 있는 공간이라고 생각합니다. 그래서 우리는 스퀴즈비츠의 탁월한 기술력이 어떻게 밝은 미래를 제시하는지, 그리고 스퀴즈비츠의 사람들이 서로를 존중하며 어떻게 성장해 나가는지 보여줄 수 있는 팀 블로그가 필요한 시점이라고 판단하게 되었습니다.